Artificial Intelligence & Machine Learning
,
Next-Generation Technologies & Secure Development
Researchers Expect an AI Training Data Drought in the Next 2 to 8 Years
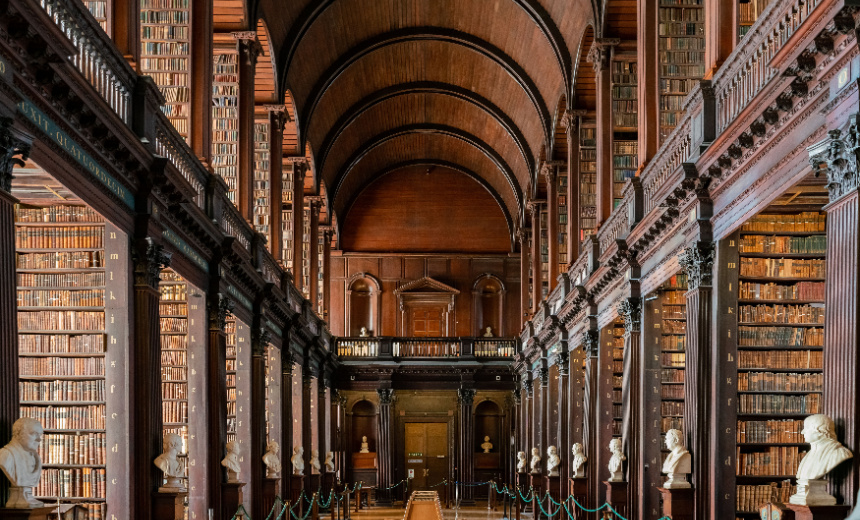
Artificial intelligence models consume training data faster than humans can produce it, and large language model researchers warn that the stocks of public text data are set to be exhausted as early as two years from now. They also say that bottlenecks aren’t inevitable.
See Also: Safeguarding Election Integrity in the Digital Age
The more data and compute AI developers use, the better the model. Boosts in compute efficiency will ensure AI models continue to improve even after available human-made training data runs dry sometime between 2026 and 2032, but likely for only a few years, said scientists from Epoch AI in a revised paper posted this month. After that, LLMs will reach a point of diminishing returns and the rate of improvement could slow down severely, said Pablo Villalobos, principal author of the study.
The amount of data AI models need depends on factors such as the complexity of the problems they’re built to solve, the model architecture and performance metrics. The Epoch paper focuses on general-purpose language models such as OpenAI’s GPT family. These models have well-known relationships, called scaling laws, between the amount of computation they require for training, the amount of data they use and capabilities of the trained model. Scaling laws say that when you increase the training compute of a model by 100, you should increase the size of its training dataset by 10, Villalobos told Information Security Media Group.
The largest published dataset used for AI training so far is Meta’s Llama 3, which used 15 trillion tokens – segments of text used to train the probabilistic output of LLMS. In English, that roughly corresponds to four characters of text. It’s possible that some closed-source models, such as Claude 3, used larger datasets.
The size of datasets for training LLMs has increased at an exponential rate of approximately 2.2 times per year, Epoch data shows. Assuming that trend continues, by 2030, models will be trained on close to a quadrillion tokens, about a hundred times more than today.
Not all training sources are alike. Training on Wikipedia text produces better models than training on the same amount of random text from the web, Villalobos said. Using unverified, crowdsourced data to train AI models, even supplementary to trustworthy information, can dilute the quality of the results – as illustrated by AI-generated responses based on training data from Reddit that advised cooks to use glue to make cheese stick to pizza better (see: Breach Roundup: Google AI Blunders Go Viral).
To ensure a more sustainable stream of training material, companies are experimenting with AI-generated training data.
OpenAI CEO Sam Altman reportedly said at a recent United Nations event that the company is already “generating lots of synthetic data” for training purposes, although he said relying too heavily on such data is inadvisable. “There’d be something very strange if the best way to train a model was to just generate, like, a quadrillion tokens of synthetic data and feed that back in,” Altman said. “Somehow that seems inefficient.”
Some researchers caution that this approach could carry significant risks of inconsistency, bias and inaccuracy unless used cautiously.
If done carelessly, this approach does not work. At best the model does not learn anything new, and at worst its mistakes are amplified, Villalobos said. “It’s like asking a student to learn by grading their own exam without any outside help or information,” he said.
But if researchers find a way to amplify the capabilities of the model, perhaps by having an external automated verification process that eliminates mistakes, it can work very well, Villalobos said.
AI-generated data is probably the only way for models to advance beyond human knowledge, he said. This was the case with the AlphaZero model, which surpassed human players in a game of Go using only synthetic data.
Villalobos drew parallels between training AI and educating human children. He said it might be worth having more autonomous AI models that can explore and interact with the real world and learn in that way, as human children do. “The fact that humans can learn in these ways indicates that it should be possible for AI models as well,” he said.
Another possibility is focusing on improving data efficiency: Humans don’t need to read trillions of words to become proficient at many tasks, so it seems that there is a lot of room for improvement, he said.
Companies could also potentially rework AI algorithms to use the existing high-quality data more efficiently. Curriculum learning is one such strategy, where training works similar to human education. Data is fed to the AI model in a particular order, in increasing levels of difficulty, to allow the model to form smarter connections between concepts, theoretically lowering the amount of new training data.
#Exhaust #Internet #Whats